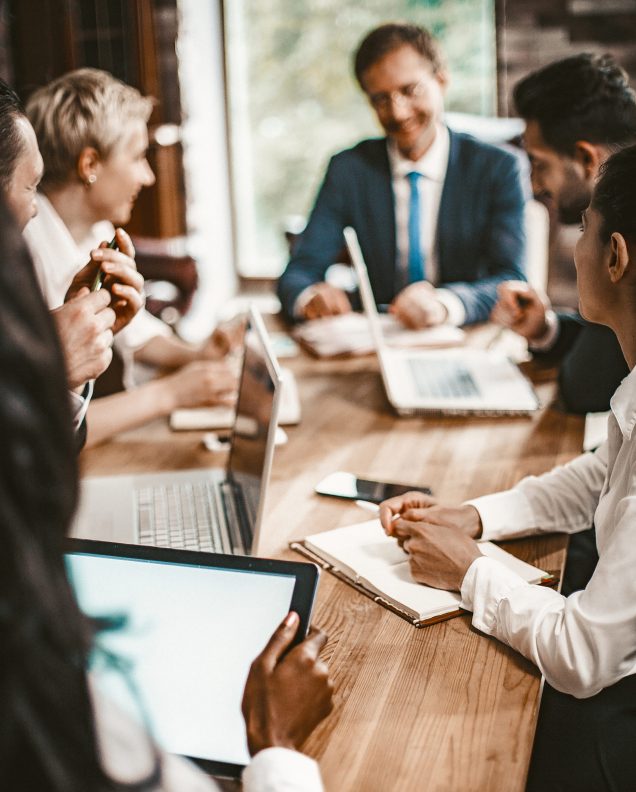
Ensuring Trust and Efficacy
20 May 2024
Blog
Artificial intelligence (AI) is being increasingly used in medicine, and one of the challenges is for AI systems to be able to understand and use different types of data. This is where multimodality comes in.
A multimodal model is a type of model that can be trained on a variety of different data types, not just text. This means that they can process and understand different modalities, such as images, videos, audio, and other sensory data, alongside text data.
These models consist of several steps that involve encoding multiple types of data into a single representation, allowing the model to process information from different sources. The models aim to learn the relationships between different modalities and have shown promise in improving language tasks and other tasks beyond what text-only models can handle.
There have been a number of recent advances in multimodal research, including:
These models all use a vision-based transformer to embed multimodal data. This suggests that a vision-based transformer could be used to handle multiple modality data related to the human body, such as MRI scans, ECG readings, genome data, X-rays, blood tests, and electronic health records (EHRs). This data combined with a vision-based approach could be used to build what we are calling a artificial medical intelligence.
AMI consists of large multimodal models that are specifically designed to handle human health data. AMI can not only process multiple modalities of data, but it will also generate these modalities as well. This means that AMI has the potential to create a multi-modal representation of the human body to help describe, diagnose, and discover.
Prevayl is currently developing its own AMI. The model is being trained initially using ECG data from open-sourced datasets. The model is made up of a combination of vision-based transformers, traditional-text based transformers and a dynamic modality mixture of experts model. All of which when brought together will output a list of sentences that will provide context to a prompt that can be passed through an LLM to generate an output that will either describe what is happening in the inputted data, diagnose a pattern that is present in the data or discover a pattern between multiple data types that has not previously been seen in labelled datasets. The initial goal is to be able to generate text outputs that can be used to determine health outcomes. Future work will look to optimise each stage of the AMI pipeline to ensure accuracy for all types of modalities related to the human body and to also allow for the generation of not only text but other modalities too.
This is an exciting area of research with the potential to revolutionise healthcare. By being able to process and understand multiple modalities of data, AI systems could be used to improve diagnosis, treatment, and prevention of diseases.